What is a type-1 error?
Consider a situation where an eCommerce business wants to improve its existing sales. They build a hypothesis that by optimizing the design of the product page they could improve the checkout rate which eventually would lead to more purchases.
To validate this hypothesis, they A/B tested the two designs and measured the click rate on the checkout button. According to the hypothesis testing approach of an A/B test, after running the experiment for a certain duration, if it declares a statistically significant result in favor of the new product page design then the new hypothesis is considered to be true.
Now suppose, the test results were wrong and truly there wasn’t any difference between the two designs, then the test has committed a False positive or Type-1 error. If an A/B or a Multivariate test declares a statistically significant result when in reality no difference exists in the performance of the variations being tested, then it is a Type-1 error.
In scientific terms, during the hypothesis testing process when a null hypothesis (representing no effect) is rejected, even if it is accurate and should not be rejected by the test then it is called a Type-1 error or a False Positive. A null hypothesis is defined before the start of an A/B test or MVT which represents no difference between the variations being tested.
To put it more formally, in an A/B test, if both variations are similar and don’t affect the metric being tested any differently, an error may occur where the null hypothesis is rejected after the test concludes. In such a case, if it’s determined that there is a statistical difference between the variations, the result is a Type I error.
Why is it important to understand type-1 errors?
After running an A/B test suppose you incorrectly conclude that variation B is a winner and deploy it for all traffic. A wrong conclusion can be detrimental to the conversion rate of a business and can lead to revenue loss. So whenever you run an A/B test, an understanding of type 1 errors can help you to
- Estimate the risk in case a wrong conclusion is made
- Perform experimentation in a scientifically disciplined manner
What causes a type-1 error?
When performing a statistical test there is always a scope of getting a type-1 error as the estimations are made over a limited sample data. A statistical test doesn’t promise to provide the right decisions every time, but the right decisions most of the time. Therefore a testing methodology must be evaluated on the basis of how well is it able to restrict the errors within a certain bound.
Type-1 errors are mainly caused due to two reasons-
- Random Chance – In a hypothesis test, an analyst uses only a small portion of the population of the data to make estimations. Therefore there exists a possibility that in certain cases the collected samples do not represent the true population leading to incorrect conclusions.
- Concluding a test early – In frequentist hypothesis testing, the testing is expected to be performed after the desired sample size needed for the study is collected. However, oftentimes the tests are ended as soon as the p-value goes below the defined threshold. This leads to an inflated false-positive rate.
Graphical representation of a type-1 error rate
The following is the representation of a null hypothesis model and alternate hypothesis model.
- The Null model represents – the probabilities of obtaining all possible results if the study were repeated with new samples and the null hypothesis were true in the population.
- The Alternate model represents – the probabilities of obtaining all possible results if the study were repeated with new samples and the alternate hypothesis were true in the population.
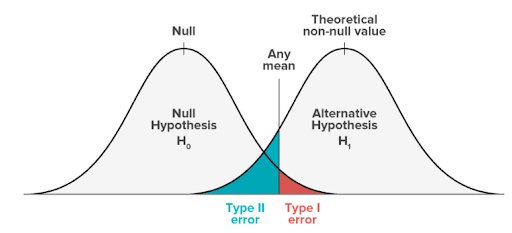
The shaded region is called the critical region. If your results fall in the red critical region of this curve, they are considered statistically significant and the null hypothesis is rejected. However, this is a false positive conclusion, because the null hypothesis is actually true in these cases.
The tradeoff between type-1 and type-2 errors
The Type I and Type II error rates affect each other in statistics. Type 1 errors depend on the significance level which affects the statistical power of a test. And the statistical power is inversely related to the Type II error rate.
This means there lies a tradeoff between the Type I and Type II errors:
- A low significance level decreases Type I error risk but increases Type II error risk.
- A high-powered test can have a lower Type II error risk but a high Type I error risk.
Type I and Type II errors occur where the distributions of the two hypotheses overlap. The red shaded area represents alpha, the Type I error rate, and the blue shaded area represents beta, the Type II error rate.
Therefore, by setting the Type I error rate, you indirectly influence the size of the Type II error rate as well.
How to control the type-1 errors?
The chance of committing this error is related to the significance level (alpha or α) you decide.
This value that you set at the beginning of your study assesses the statistical probability of obtaining your results (p-value). P-value is a term majorly used in frequentist statistics.
As per academic literature, the significance level is usually set at 0.05 or 5%. It means out of 100 tests where variations are the same, 5 tests will say variations are statistically different. If the obtained p-value of your test is lower than the configured significance level, it means the difference is statistically significant and consistent with the alternative hypothesis. However, the difference is statistically non-significant if the p-value is higher than the significance level.
To reduce the Type I error probability, you can simply set a lower significance level and run experiments longer to collect more data.
At VWO, we use Probability to be the Best (PBB) and Absolute Potential Loss (PL) as the decision-making metrics to determine a winning variation. The use of PL metric with PBB ensures that even if a type-1 error occurs, the overall impact of the wrong decision is tolerable by the business.
To understand more about how exactly VWO can help you bring down such errors, try out VWO’s free trial or request a demo by one of our optimization experts.